Many of us have experienced the shock of an electric bill that exceeds our expectations—and our budgets. At home, we can respond by turning lights off when not in use, switching to energy-efficient bulbs, and other common ways of reducing energy consumption.
But what if we had to continue our daily activities without these options? Predicting energy costs and creating a realistic budget would become a top priority. That’s exactly the predicament many manufacturers face: in the absence of ways to reduce electricity use while still meeting production goals, they aim to accurately forecast usage so they can make informed financial decisions.
This was the approach taken by a semiconductor plant in Thailand, which used Minitab Statistical Software to examine its fluctuating electrical load. The production of semi-conductive material, which is the foundation for many electronics, is in high demand and the plant’s equipment must run almost continuously. Using data analysis, a project team at the plant set out to determine the most precise electricity consumption forecasting method in order to maximize profits.
The Challenge
Forecasting models identify patterns in data and then use those patterns to predict what a certain variable—in this case, the plant’s electricity consumption—will do in the future. The team needed to analyze three years of historical data from the Metropolitan Electricity Authority (MEA) using six established forecasting models and one new method created for this project. The new method incorporates factors specific to the plant’s semiconductor manufacturing process.
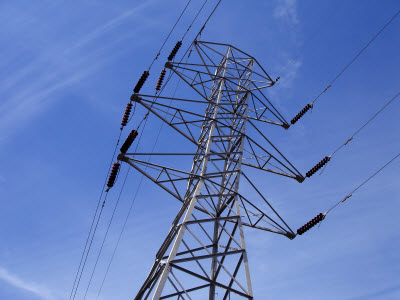
A project team used Minitab Statistical Software to analyze data that would determine the best method for electricity consumption and reduce costs.
Each forecasting method would produce predictions for the plant’s electrical load six months into the future. These results could then be compared to historical data. By calculating the Mean Absolute Percentage Error (MAPE), which expresses the accuracy of a particular forecasting method as an error percentage, the team could then determine which model provides the most accurate predictions.
The project team needed to evaluate each method and use the model with the lowest MAPE value in order to assess the cost of electricity, create a sensible budget, and make sound financial decisions.
How Minitab Helped
Organization
Semiconductor plant in Nonthaburi, Thailand
Overview
- Founded in 1984
- Employs over 1,300
- Operations include assembling and testing Flash memory products
Challenge
Select the best electricity consumption forecasting method in order to reduce costs and assist in decision making
Products Used
Minitab® Statistical Software
Results
- Determined the best method for predicting electricity consumption six months into the future
- Reduced costs of electrical consumption
- Established a new method that can be applied to other plants/businesses